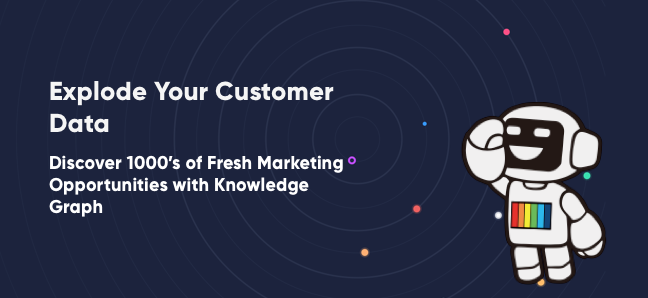
I wanted to use our own tech and show that you can cross-reference your sales data with the 10+ billion entities stored in Diffbot Knowledge Graph, to find marketing opportunities with a little #KnowledgeHack. I wasn’t disappointed with what I found.
Because the Diffbot Knowledge Graph (KG) focuses on people, companies, and location data, I wanted to see how it could help me target the right people with a timely message via one of the major ad platforms like Facebook, AdWords, or LinkedIn.
This “how-to” guide shows you, step by step, how I used the Diffbot Knowledge Graph to explode a few of our best customers’ data into a list of thousands of high-value marketing targets in just a few steps:
- Take a small number of existing customers.
- Define an Ideal Customer Profile (ICP) based on their common attributes and connections.
- Find every person and/or business online who matches that profile.
- Analyze those people as a group, and build a marketing campaign with the insights.
Caveats
- This is not a silver bullet, and requires some critical thinking on your behalf, following this guidewill give you useful data, it wont do your marketing for you.
- You will need a Diffbot Knowledge Graph (DKG) account to do this. The whole technique revolves around using the vast amount of people and company data stored in the DKG, and its ability to search through their connections to get results.
Step One
Define an ideal customer profile (ICP) for a campaign based on your own customers.
Find a few examples of your best customers.
To find them, simply ask your sales team who the best customers or leads are, or run a report in your CRM to show you your top existing customers.
E.g.: Run an “All Closed Won by Revenue” or, even better, “All Closed Won by LTV” report.
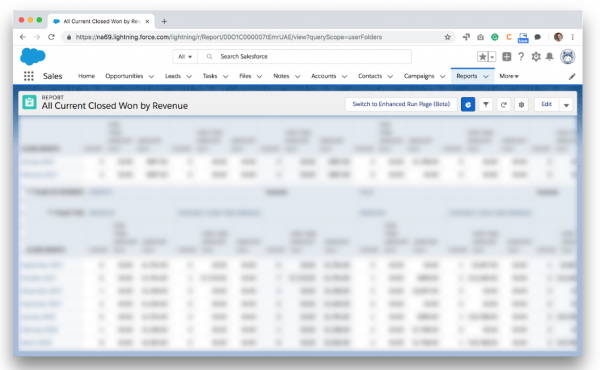
That will give you the names and locations of several example people you can use to create a template to find other similar (look-alike) candidates.
For this guide, I decided to use made up existing customers by looking up some example profiles I found by searching for “People who are currently employed as ecommerce managers at companies with more than 300 employees.” You can see the query for this example below:
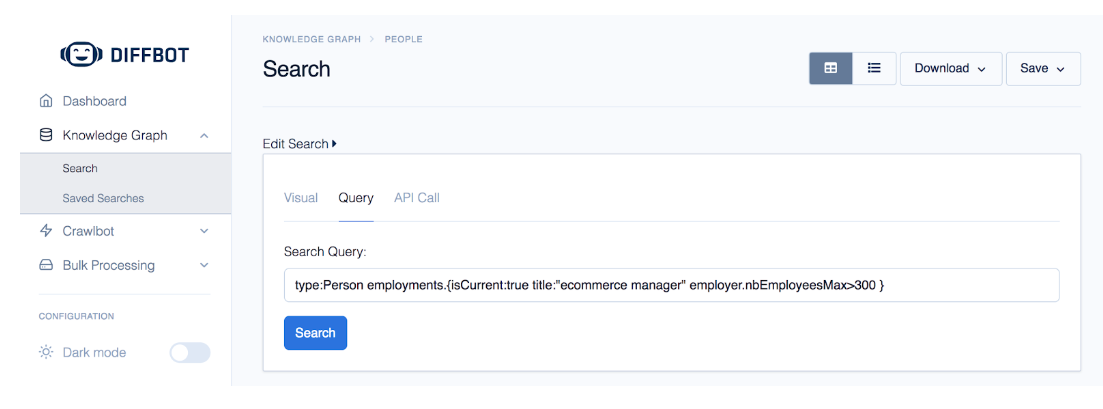
The query above basically filters for type=person, current employment job title = “ecommerce manager,” and their current employer has more than 300 employees. Don’t worry too much about the search query and how to make those right now; there are lots of guides and documentation during onboarding that show you how easy it is. For now, just imagine it’s like making filters in Excel or Google Sheets.
That search gives some results you can substitute in place of actual existing customers.
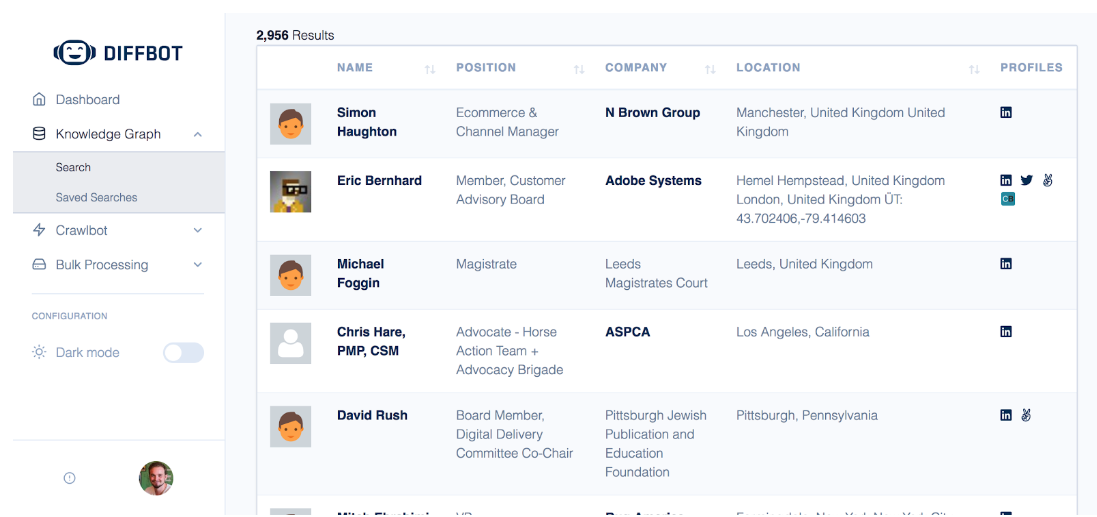
Step Two
Explode a few prime example customers into thousands of similar potential customers.
Once you have your existing (or made up — see above) customer profiles, you can find them in the KG with a simple query like this:
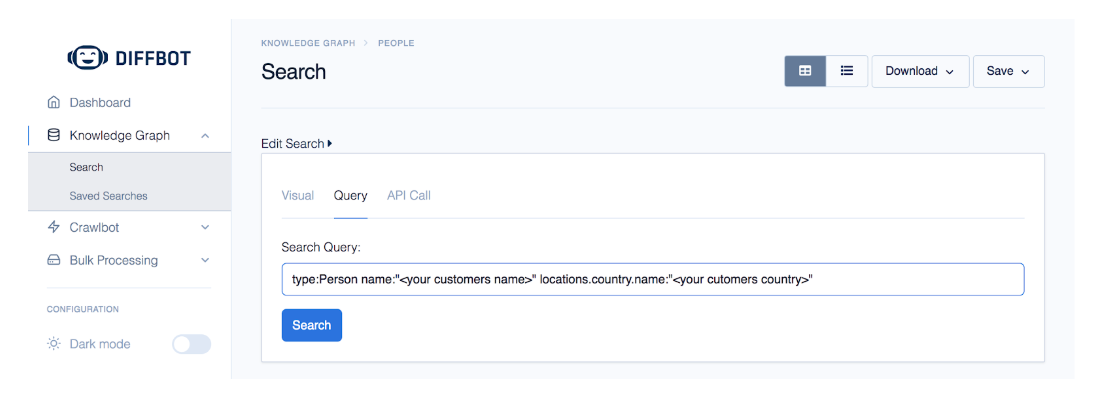
And view their information by clicking on their profile from the results:
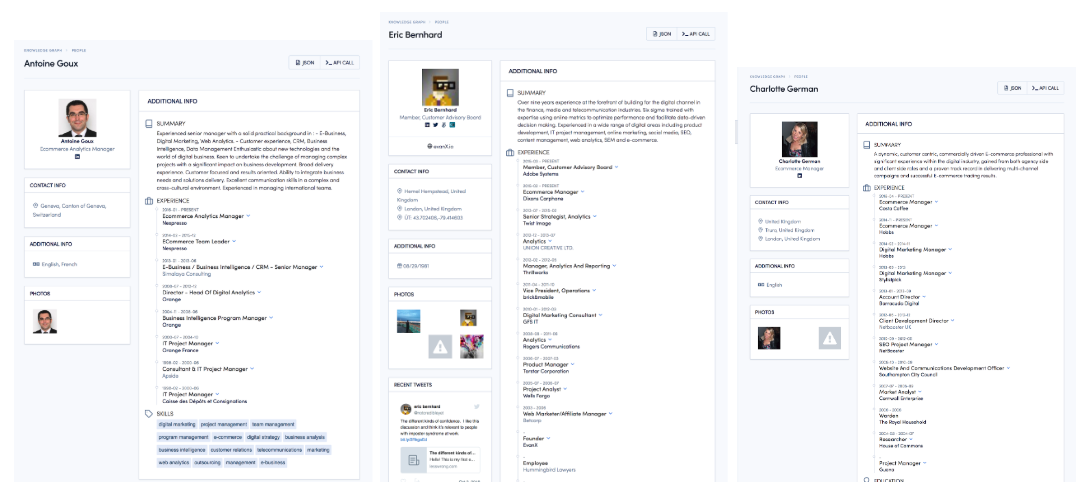
You will quickly begin to spot commonalities between the profiles. Excuse the crude visualization, but it will look something like this:
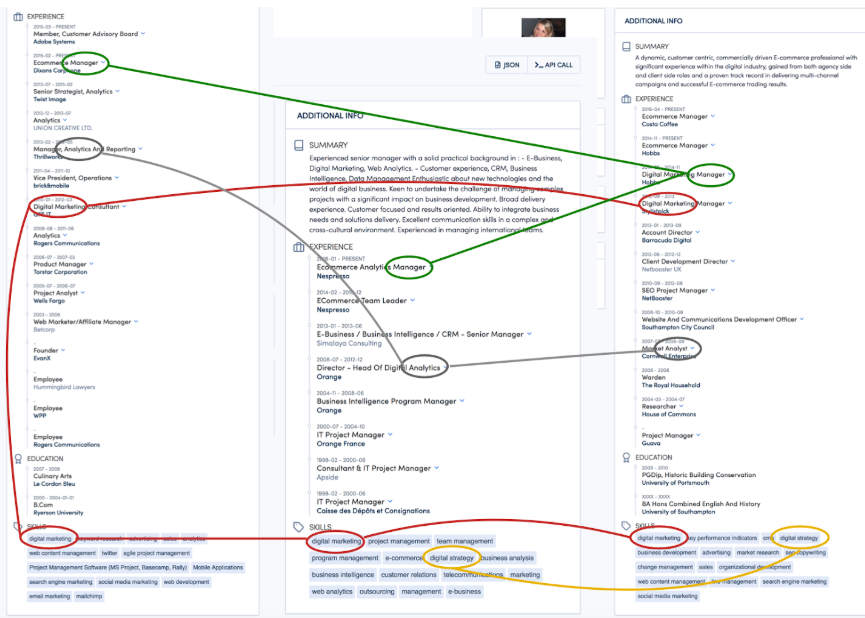
In this example, you can see several similarities between your existing customers.
- Job title
- Skills
- Experience
- Education
- Industries
And you can do the same with the employer’s profiles, too.
Click through to see the people and employers to compare and contrast for similarities.
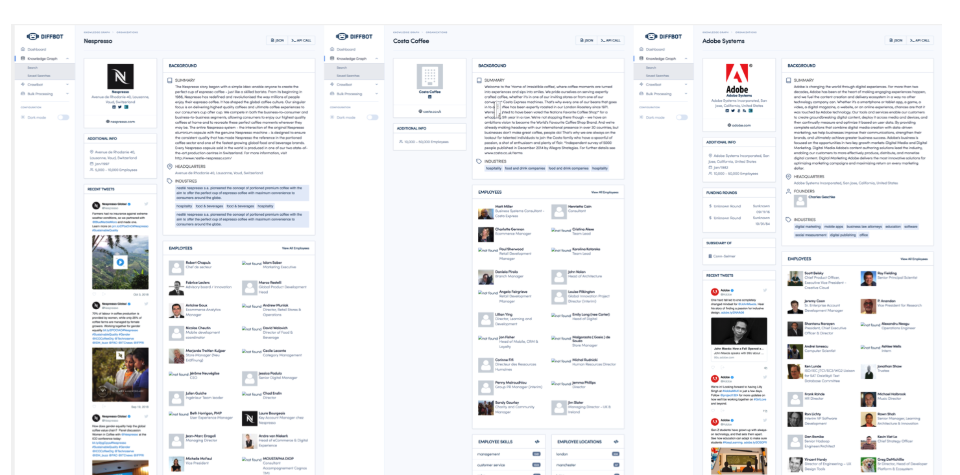
In this case, the companies of the example customers I found have no less than 5,000 employees, and all use jQuery as a front-end technology. At first, that might seem irrelevant, but here comes the good bit…
You can use those common attributes to find more people just like them, to create a look-alike audience on the web scale. How?
Build a query that looks for those common attributes, like this example:
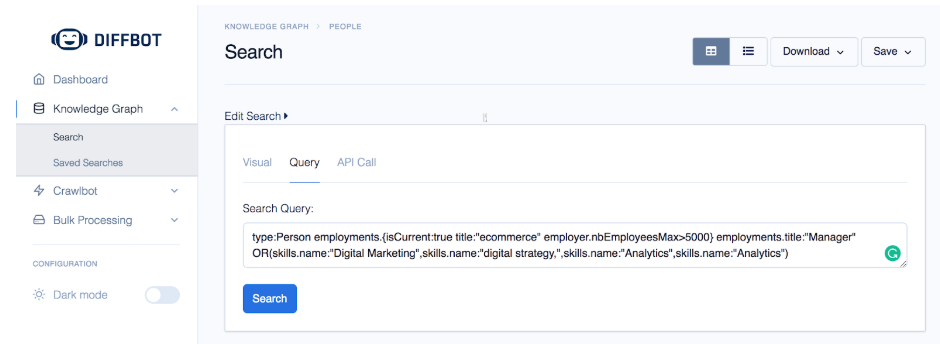
- Skills: Digital Marketing, digital strategy, Analytics
- Current Job titles: ecommerce
- Past job titles: Manager
- Locations: Major cities
- Current Employer Company size: 5,000+
- Current employer location city size: 100,000+
- Current Employer Technology Used: jQuery
Hooray! That query returns 2,363 people. (at time of writting)
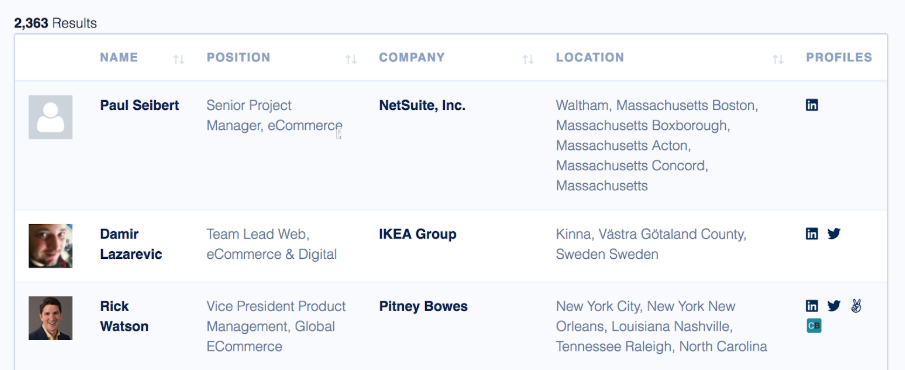
That is a list of all the people who are a good likeness for your Ideal Customer Profile. Perfect! Of course, you will need to check the data and remove any people who don’t meet your particular needs, but in general, you have a great dataset to start working with.
Any good salesperson or marketer will know several ways to use that data to generate demand and leads from that market.
- You can use their social media information to reach out to them with a tweet or message.
- You can target ads at these people and organizations via LinkedIn, Facebook, and other platforms.
- Use other data enrichment tools such as Pipl to learn even more about those people.
- You can invite them to your events, webinars, and other engagement platforms.
But what to say to them?
In this case, we know the following about them:
- They work in large organizations
- in major cities
- doing management in and around digital marketing for companies.
- They often use jQuery and other similar front-end technologies.
- Your existing customers’ use cases are likely to be relevant.
For Diffbot, that may well mean that we:
- Write a “how to” blog post about how to use Diffbot to help them do something cool in marketing.
- Sponsor and/or attend local events about digital marketing, and evangelize our Knowledge Graph in context of their needs.
However, I wanted to take it a step further and learn more about these people using the Knowledge Graph to build a better picture of the market. To do that, I started segmenting and grouping the data using some advanced Knowledge Graph features.
Bonus Step Four
Analyze the group of people who match my ICP for further insights.
Here are some basic things you can learn:
“Who are the companies that currently employ this type of person the most?”
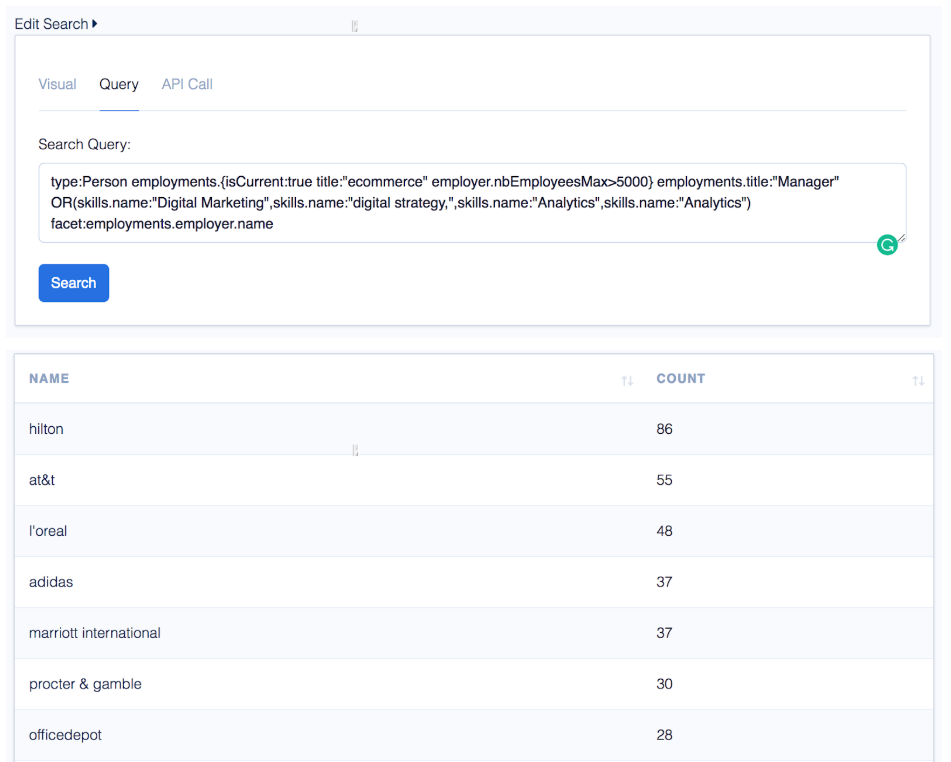
“What are the descriptors of companies that currently employ this type of person the most?”
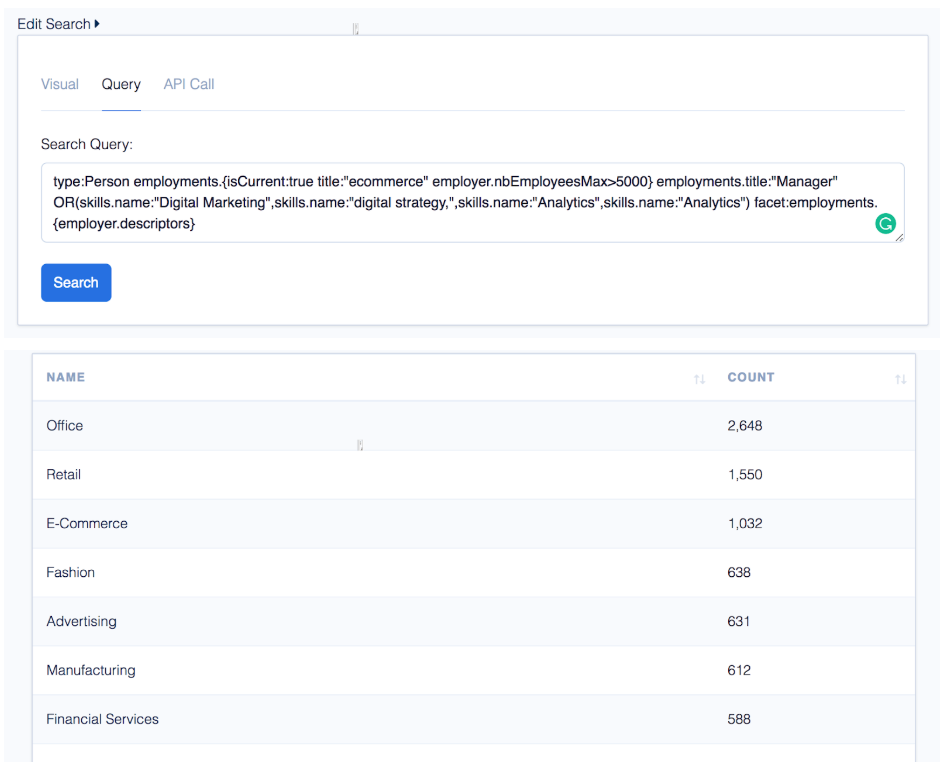
“What is the gender split of this type of person?”
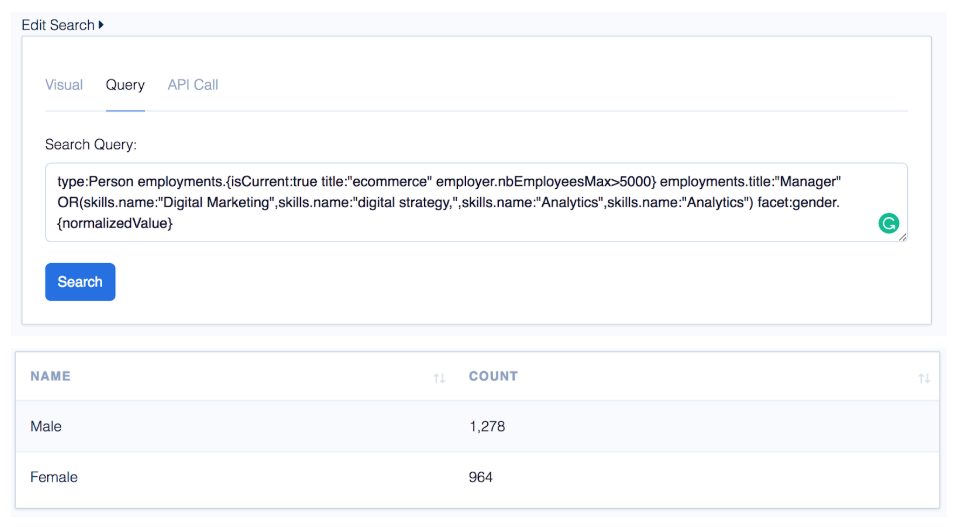
“What is the location split of this type of person?”
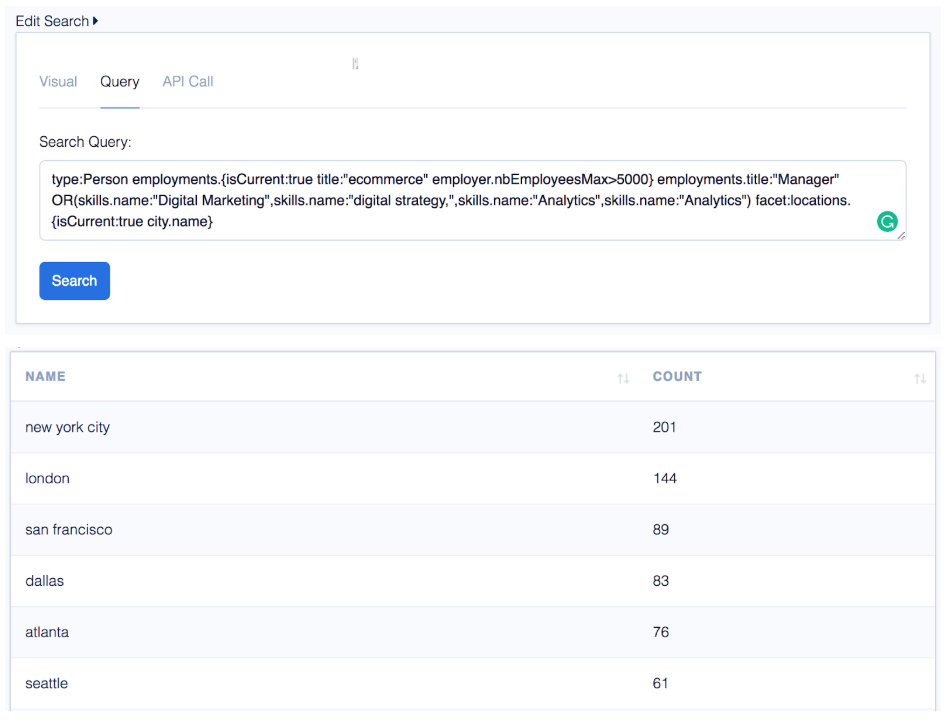
Now you’re armed with Data.
Now that you are armed with the data you need, you can tailor your marketing activity to match the audience gender, location, and employer type. And don’t forget, you have a list of 2,600+ leads from earlier in the process.
Off the back of this research, we are now considering how we can target those customers with some interesting, intelligent, and high-value marketing activity — perhaps joining digital marketing and ecommerce Hackathons in those locations. Perhaps writing some API script templates in jQuery? Perhaps simply answering questions on Stack-overflow relating to marketing and ecommerce data!
Rinse and repeat for your different customer segments, and you will have all the insights you need to grow your business.
Try this technique for yourself
To try this technique for yourself, you do need access to Knowledge Graph, which you can request here. If you have any questions please leave comments below.
You must be logged in to post a comment.