8 Ways Web-Reading Bots Are Revolutionizing Market Intelligence
Join us on July 29th as we work through ways in which Diffbot’s Knowledge Graph and Natural Language API provide you with the power of “web-reading” bots for market intelligence tasks. Featured on this webinar includes a high-level view of how to effectively evaluate market monitoring solutions as well as hands-on market monitoring techniques. Check out the on-demand webinar here.
Best Practices: Using External Data To Enrich Internal Databases
Join us on July 22nd as we showcase the risks of letting data decay over time without a solid plan for data enrichment. In this hands-on presentation we work through how bulk Enhance (Diffbot’s data enrichment product) works, the power of linked data, and how our Enhance-enabled integrations work. Register for this on-demand webinar here.
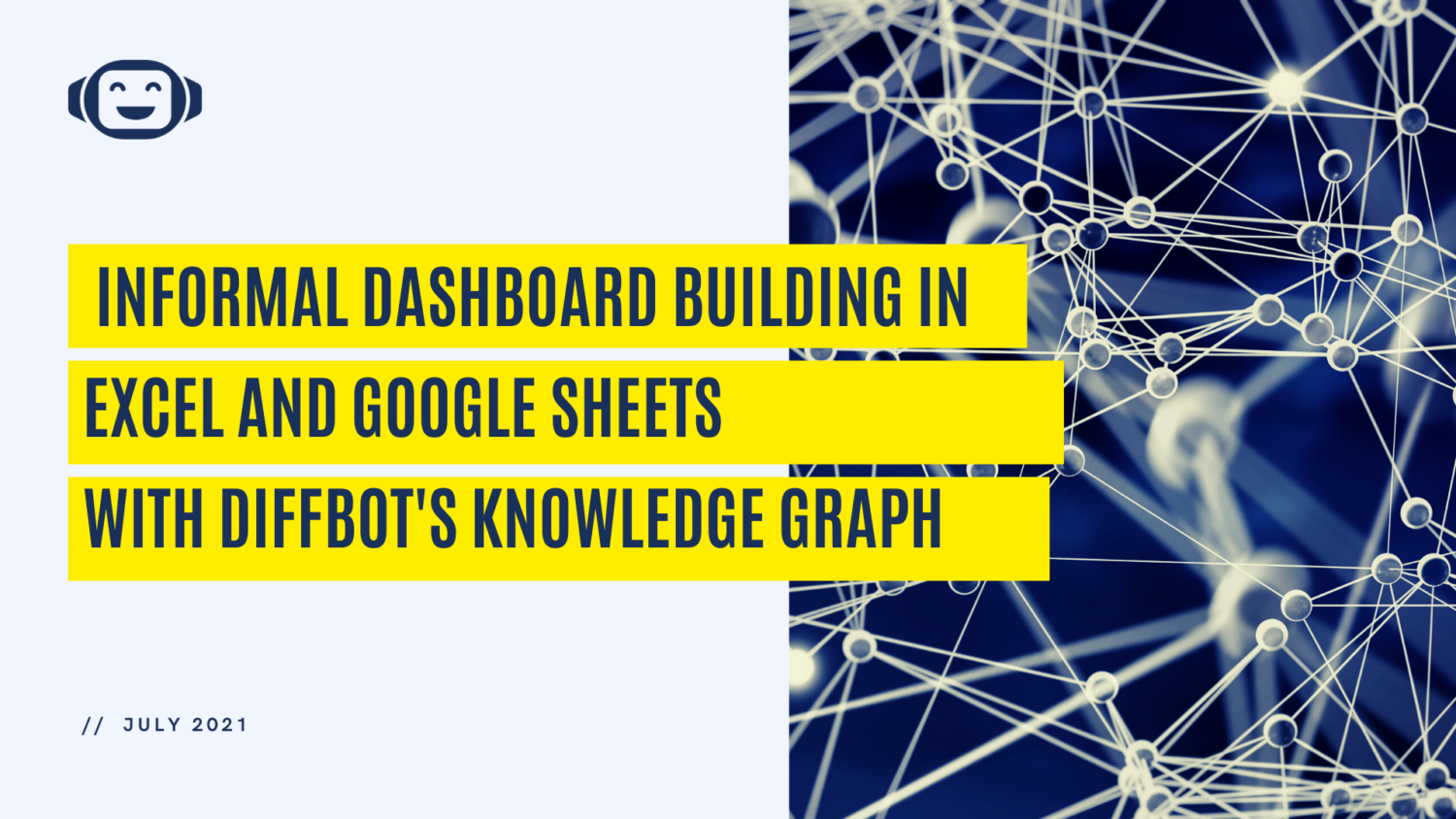
Informal Dashboard Building With Diffbot’s Excel and Google Sheets Integrations
Join us July 1st, as we pull in data from the world’s largest Knowledge Graph directly into Google Sheets and Excel. This webinar centers around creating the “building blocks” of external data dashboards within spreadsheets. Register for this on-demand webinar here.
Knowledge Graph Techniques For Global News Monitoring
Join us June 24th as we work through some of the top ways in which we’re seeing our Knowledge Graph news index (50x the size of Google’s) utilized to build structured article data feeds and dashboards. Register for this on-demand webinar here.
Competitor, Vendor, And Customer Data From Across The Web With Diffbot’s Knowledge Graph
Join us June 17th as we explore ways in which the world’s largest Knowledge Graph is a great fit for market intelligence data from all over the web. Register for this on-demand webinar here.
[E3] 4 Ways Technical Leaders Are Using Natural Language Processing To Drive Data Transformations
Join us June 10th as we explore ways in which Natural Language Processing is promoting data transformations in ecommerce, human resources, market intelligence, and news monitoring. Register for this on-demand webinar here.
[E2] How To Wrangle The Entire Web Into A Valuable Media Monitoring Dashboard
In this webinar we work through the three “building blocks” of structured public web data that enables world-class media monitoring dashboards. Register for this on-demand webinar here.
[E1] Are Rules Meant To Be Broken? Rule-based Versus Rule-Less Web Data Extraction
Humans don’t follow a rigid set of rules when we read the web. So why should web data extraction bots? Register for this on-demand webinar here.
Other Events
DataBytes Netcast Episode 4 With Ganes Kesari
In this DataBytes we talk with co-founder and chief decision scientist at Gramener. Ganes steps viewers through a key issue he sees between the relationship between data and decision-making teams: that they don’t decide on a business problem to solve before deciding on a data problem.
DataBytes Netcast Episode 3 With Doug Laney
In this DataBytes we talk with bestselling author, thought leader, and professor Doug Laney on why he often sees data teams fail to treat their data as an asset.
DataBytes Netcast Episode 2 With Praveen Balaji
In this DataBytes netcast senior architect at Diffbot Praveen Balaji talks about how we can take the hype out of machine learning. This leads the way to a much wider array of software engineers integrating ML tooling and techniques into their workflows.
DataBytes Episode 1 With Julia Wiedmann
In our inaugural DataBytes netcast we talk with knowledge graph researcher at Diffbot, Julia Wiedmann. She details how important a focus on stepping back and assessing bias in ML training data can be. Additionally, Julia talks through the importance of end-to-end machine learning.
You must be logged in to post a comment.