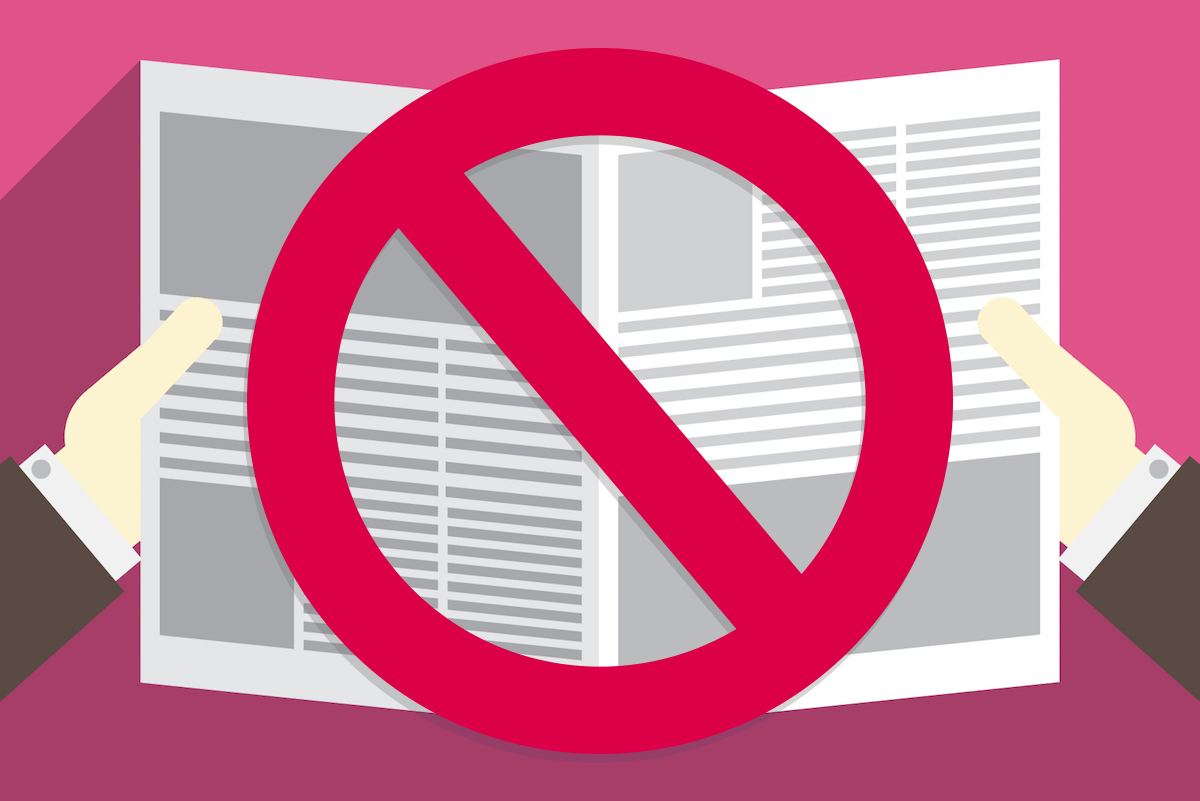
Any developer who has ever attempted to build their own scraper has probably asked the question, “Why is this so damn hard?”
It’s not that building a scraper is challenging – really anyone can do it; the challenge lies in building a scraper that won’t break at every obstacle.
And there are plenty of obstacles out there on the web that are working against your average, homegrown scraper. For instance, some sites are simply more complex than others, making it hard to get info if you don’t know what you’re looking for.
In other cases, it’s because sites are intentionally working to make your life miserable. Robust web scrapers can usually overcome these things, but that doesn’t mean it’s smooth sailing.
Here are a few of the biggest reasons that getting data from the web – particularly clean article data and product data – is so incredibly frustrating.
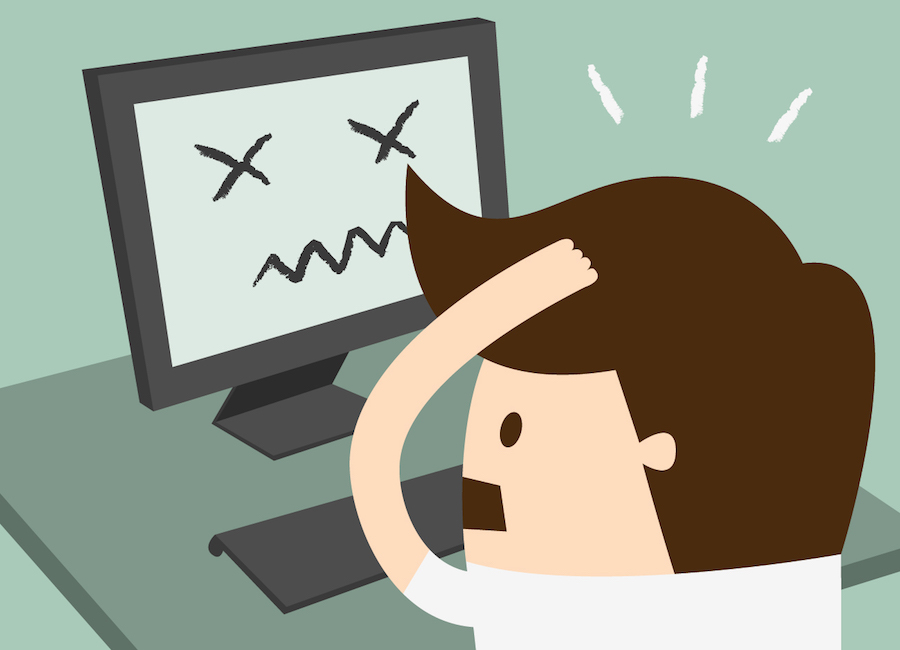
The Web Is Constantly Changing
If there’s one thing that can be said about the web, it’s that it’s in constant flux. Information is added by the second, websites are taken down, removed, updated and changed at break-neck speeds.
But web scrapers rely on consistency. They rely on recognizable patterns to identify and pull relevant information. Trying to scrape a site amidst constant change will almost inevitably break the scraper.
The average site’s format changes about once a year, but smaller site updates can also impact the quality or quantity of data you can pull. Even a simple page refresh can change the CSS selectors or XPaths that web scrapers depend on.
A homegrown web scraper that depends solely on manual rules will stop working if changes are made to underlying page templates. It’s difficult, if not impossible, to write code that can adjust itself to HTML formatting changes, which means the programmer has to continually maintain and repair broken scripts.
Statistically speaking, you will most likely have to fix a broken script for every 300 to 500 pages you monitor, but more often if you’re scraping complex sites.
This doesn’t include sites that use different underlying formats and layouts for different content types. Sites like The New York Times or The Washington Post, for example, display unique pages for different stories, and even ecommerce sites like Amazon constantly A/B testing page variations and page layouts for different products.
Scrapers rely on rules to gather text, looking at things like length of sentences, frequency of punctuation, and so on, but maintaining rules for 50 pages can be overwhelming, much less 500 (or 1,000+).
If a site is A/B testing their layouts and formats, it’s even worse. Ecommerce sites will frequently test page layouts for conversions, which only adds to the constant turnover of information.
Sites won’t tell you what’s been updated, either. You have to find the changes manually, which can be hugely time-consuming, especially if your scraper is prone to errors.
Sites Are Intentionally Blocking Scrapers
On top of that, you have to worry about sites making intentional efforts to stop you from scraping data. There is plenty that can be done to halt your scraper in its tracks, too.
Sites often track the usage of anonymous users, for example, using browser fingerprinting.
If your scraper visits a page too many times or too quickly, it may get banned. Even if it’s not outright banned, a site can also hellban you, making you a sort of online ghost: invisible to everyone but yourself.
If you’re hellbanned, you may be presented with fake information, though you won’t know it’s fake. Many sites do this intentionally, creating a “honeypot,”, pages with fake information designed to trick potential spammers.
They may also render important information in JavaScript, which many scrapers can’t support.
Another of the biggest obstacles to scraping ecommerce sites is software like reCAPTCHA. A typical CAPTCHA consists of distorted text, which a computer program will find difficult to interpret, and is designed to tell human users and machines apart.
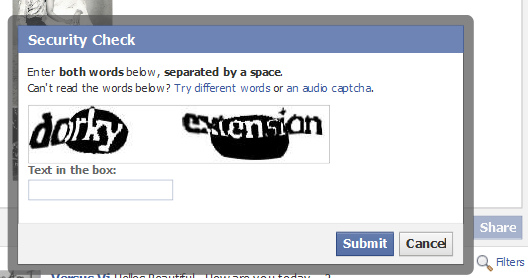
Source: Flickr
CAPTCHAs can be overcome, however, using optical character recognition (also known as optical character reader, OCR), if the images aren’t distorted too much (and images can never be too distorted, otherwise humans will have trouble reading them, too).
But not every developer has access to OCR, or knows how (or has the ability) to use it in conjunction with their web scrapers. A homegrown web scraper most likely won’t have the ability to beat CAPTCHAs on its own.
That’s not even the only obstacle that scrapers face. You might also encounter download detection software, blacklists, complex JavaScript or other code, intentionally changed markup or updated content, I.P. blocking, and so on.
Larger and well-developed web scrapers will be able to overcome these things – like using proxies to hide IP addresses from blacklists, for example – but it takes a robust tool and a lot of coding to do it, with no guarantees of success.
Some websites may do things unintentionally to block your efforts, too. For example, different content may be displayed on a web page depending on the location of the user.
Ecommerce stores often display different pricing data or product information for international customers. Sites will read the IP location and make adjustments accordingly, so even if you’re using a proxy to get data, you may be getting the wrong data.
Which leads to the next point…
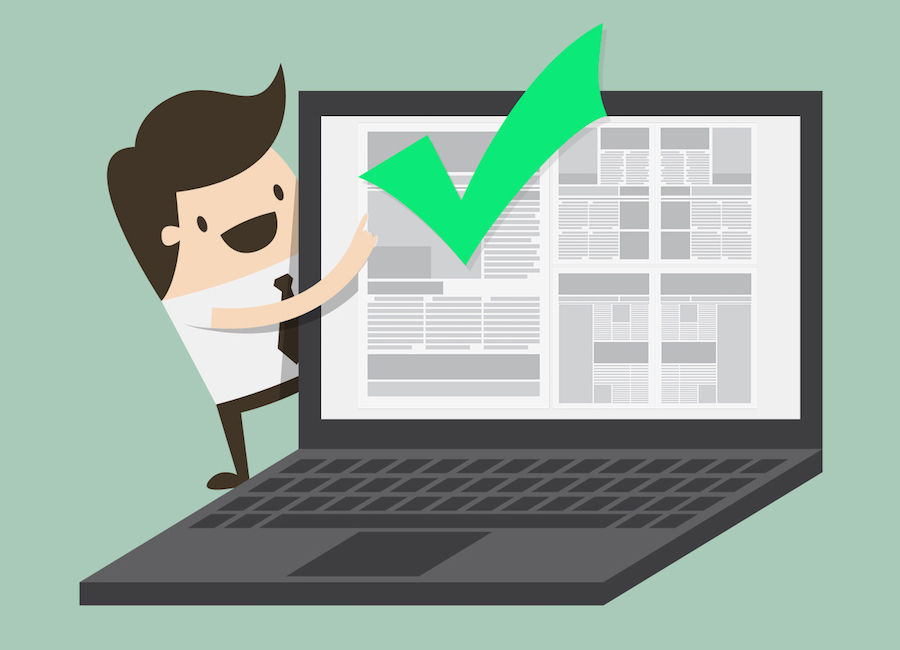
You Can’t Always Get Usable Data
A homegrown web scraper can give you data, but the difference in data quality between a smaller scraper and a larger, automated solution can be huge.
Both homegrown and automated scrapers use HTML tags and other web page text as delimiters or landmarks, scanning code and discarding irrelevant data to extract the most relevant information. They both can turn unstructured data into .JSON, .CSV, XLS, .XML or other form of usable, structured data.
But a homegrown scraper will also have excess data that can be difficult to sort through for meaning. Scraped data can contain noise (unwanted elements that were scraped with the wanted data) and duplicate entries.
This requires additional deduplication methods, data cleansing and formatting to ensure that the data can be utilized properly. This added step is something you won’t always get with a standard scraper, but it’s one that is extremely valuable to an organization.
Automated web scraping solutions, on the other hand, incorporate data normalization and other transformation methods that ensure the structured data is easily readable and, more importantly, actionable. The data is already clean when it comes to you, which can make a huge difference in time, energy and accuracy.
Another thing that automated solutions can do is target more trusted data sources, so that information being pulled is not only in a usable format, but reliable.
Final Thoughts
Getting clean data from the web is possible, but it comes with its own set of challenges. Not only do you have to overcome the ephemeral nature of the web, some sites go out of their way to ensure that they change often enough to break your scrapers.
You also have to deal with a bevy of other barriers, like CAPTCHAs, I.P. blocking, blacklists and more. Even if you can get past these barriers, you’re not guaranteed to have real, usable, clean data.
While a homegrown web scraper may be able to bypass some of these challenges, they’re not immune to breaking under the pressure, and often fall short. This is why a robust, automated solution is a requirement for getting the most accurate, clean and reliable information from the web.
You must be logged in to post a comment.