Enough individuals have heard the siren song of Robotic Process Automation to build several $1B companies. Even if you don’t know the “household names” in the space, something about the buzzword abbreviated as “RPA” leaves the impression that you need it. That it boosts productivity. That it enables “smart” processes.
RPA saves millions of work hours, for sure. But how solid is the foundation for processes built using RPA tech?
Related Reads:
- Comparison of Web Data Extractors: Import.io vs. Diffbot
- Is RPA Tech Becoming Outdated? Process Bots vs. Search Bots
- Advanced Crawlbot Tutorial: Crawling Behind Logins
First off, RPA operates by literally moving pixels across the screen. Repetitive tasks are automated by saving “steps” with which someone would manipulate applications with their mouse, and then enacting these steps without human oversight. There are plenty of examples for situations in which this is handy. You need to move entries from a spreadsheet to a CRM. You need to move entries from a CRM to a CDP. You need to cut and paste thousands or millions of times between two windows in a browser.
These are legitimate issues within back end business workflows. And RPA remedies these issues. But what happens when your software is updated? Or you need to connect two new programs? Or your ecosystem of tools changes completely? Or you just want to use your data differently?
This shows the hint of the first issue with the foundation on which RPA is built. RPA can’t operate in environments in which it hasn’t seen (and received extensive documentation about).
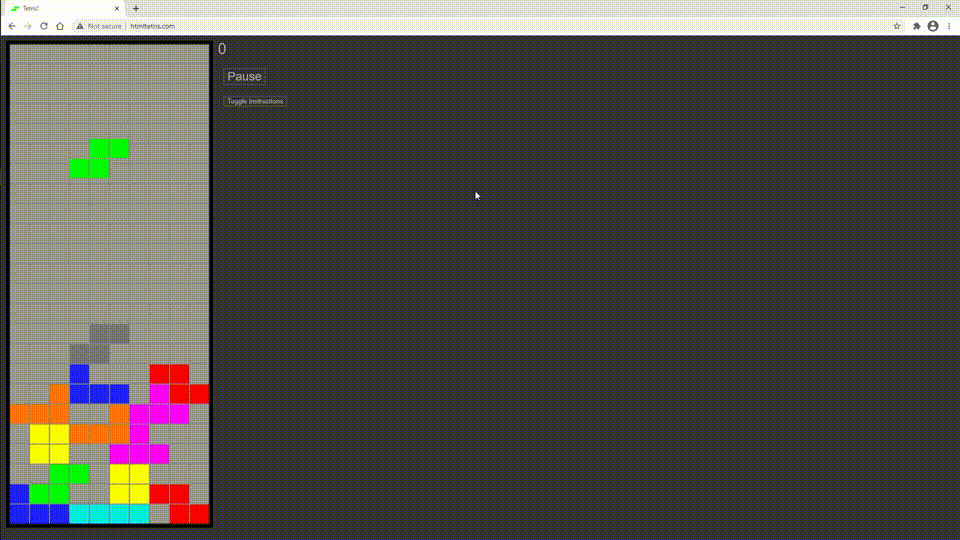
This is an issue we’ve spent a great deal of time working at Diffbot, where our web extraction tech needs to be able to extract data from pages without knowing their structure in advance.
The crux of this disconnect can be explained in rule-based versus ruleless data extraction. Rule based extraction takes time to “train” (aka specify which steps should be taken) and only works when environments are unchanged. Something as simple as a small visual tweak to an application or site can break rule-based automation and extraction.
And most data extractors — even if named to the contrary — are still rule-based extractors.
More generally, rule-based automation is also prone to failure on the input front. Nearly every enterprise solution provides programmatic or bulk manual routes to input information in a structured way. These input routes are built for maximum reliability. And circumventing these routes means you’re stuck with troubleshooting and support on your end.
This leads to the second issue with RPA. That RPA doesn’t build processes that promote general interoperability among apps.
By building data pipelines in the form of structured data — rather than a sequence of human-like interactions — your data is freed to move from database to database, or from tool to tool. Your ability to do anything at all with your data is typically a function of what tool you’re processing it with. Why would you limit yourself to the current or future capabilities of a given tool?
While you’re at it, why even restrict yourself to your data? Interoperability-proofed data can easily be merged, enriched, or transformed; whether by data teams or through the interfaces of the apps you use. This is a third issue with RPA, RPA deals with data “as it is.”
You may have heard of intelligent applications. In short, intelligent applications rely on not only dealing with the presentation of data. But in being able to make decisions based on what the data means. The data has context, and a type. Based on an ontology, facts can be derived about entities. This magnifies the value of data immensely. Rather than merely searching through strings of characters, semantic data enables you to manipulate objects like they’re “things.”
Intelligent applications are a byproduct of something like the vision of a fully-semantic web. And they’re true value comes from data that’s interoperable and has context.
A vast majority of information on the public web or within enterprise applications isn’t semantic. A vast majority of this data isn’t even structured in a form applications can use it in the first place.
So if you’re going to go through the expense and trouble to port data into a business system. Why not make it semantic at the same time?
Extraction tools built around machine vision and natural language processing can understand the data and documents they’re parsing. That’s how Diffbot’s Knowledge Graph, Natural Language API, and extraction products are able to attach elements like sentiment scores, or determine the topic of or speakers in an article.
Finally, RPA is productized in an archaic way. In our data-inundated lives, you shouldn’t have to pay thousands of dollars for a bespoke replacement of a manual task (that doesn’t even free your data in the end anyway).
Sure, thousands of dollars spent for a “process bot” saves money compared to the human cost. But that says nothing about the technical debt RPA can set you up for. In a time when underutilized data is more of a threat than lack of data, interoperability and providing context for data should be paramount in any data-driven organization’s objectives.
Using Diffbot Web Extraction To Build Future-Facing Automations
At a high level we’ve looked at some the pros and cons of RPA for data extraction and app integration creation. So how does Diffbot fit into the picture?
First, our Crawlbot product extract data behind logins. Use one of our rule-less Automatic Extraction APIs for many content types, or a Custom API for extracting any data of your choice.
Second, the structured data from within your own app can be enriched or augmented. Enhance, our data enrichment product draws from our Knowledge Graph to provide contextual information about organizations and people. Our Knowledge Graph is the largest of its type and can be used to augment your data stores with linked data about organizations, people, and an article index 50x the size of Google News. Want to create your enrichment integration fast? Check out Diffbot Enhance in Zapier.
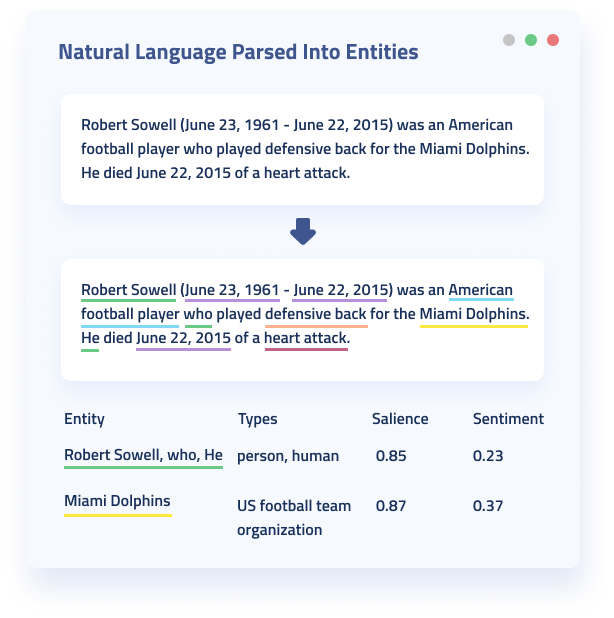
Third, mine your internal data for insights with our Natural Language API. Best-in-class entity recognition and sentiment can take your existing data and build out valuable linked data for market intelligence, news monitoring, and general recognition of trends.
What next?
Enjoy freed interoperable data enriched by NLP and augmented by structured data from across the web!
Interested in learning how Diffbot’s web data extraction products fit into your knowledge pipeline? Contact our data services team or set up a free 14-day trial today!
You must be logged in to post a comment.