Skip Ahead
In the 2002 movie Time Machine, Dr. Alexander Hartdegen, played by Guy Pearce, invents a time machine and travels forward in time to 2030. He stops by the New York Public Library and meets Vox-114, a holographic library assistant who is “connected to every database on the planet”. Vox-114 retrieves and summarizes facts conversationally, with a simple wave of a holographic hand. He even insists that time travel is not possible!
Well, we’re finally here with 7 years to spare. ChatGPT does it all, including the same reference Vox made to fiction when asked about time travel, but decidedly less tongue in cheek.
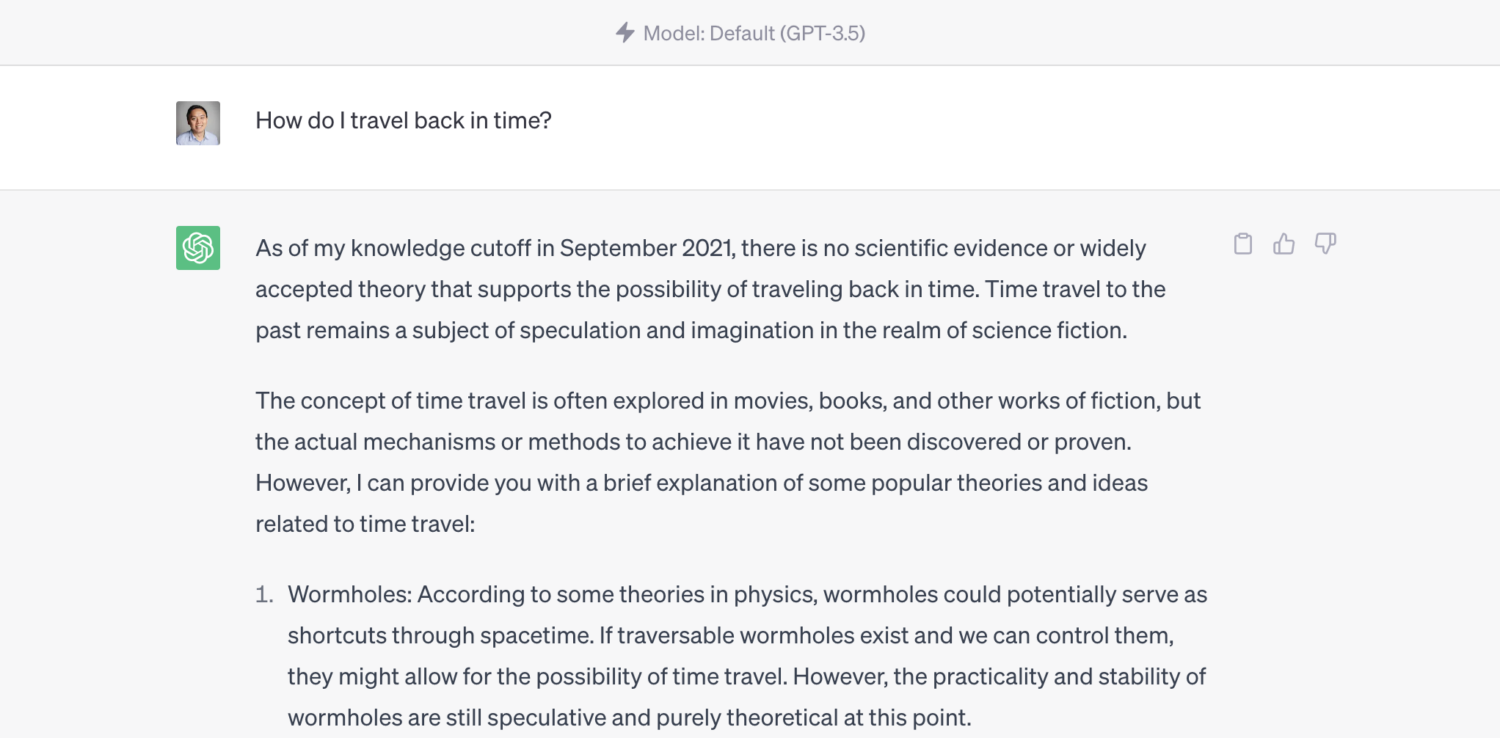
Except that ChatGPT is not “a compendium of all human knowledge”. It’s a language model trained on gargantuan stores of human knowledge to predict next word associations with human-like conversational precision.
Let’s try a contrived example using GPT-3.5
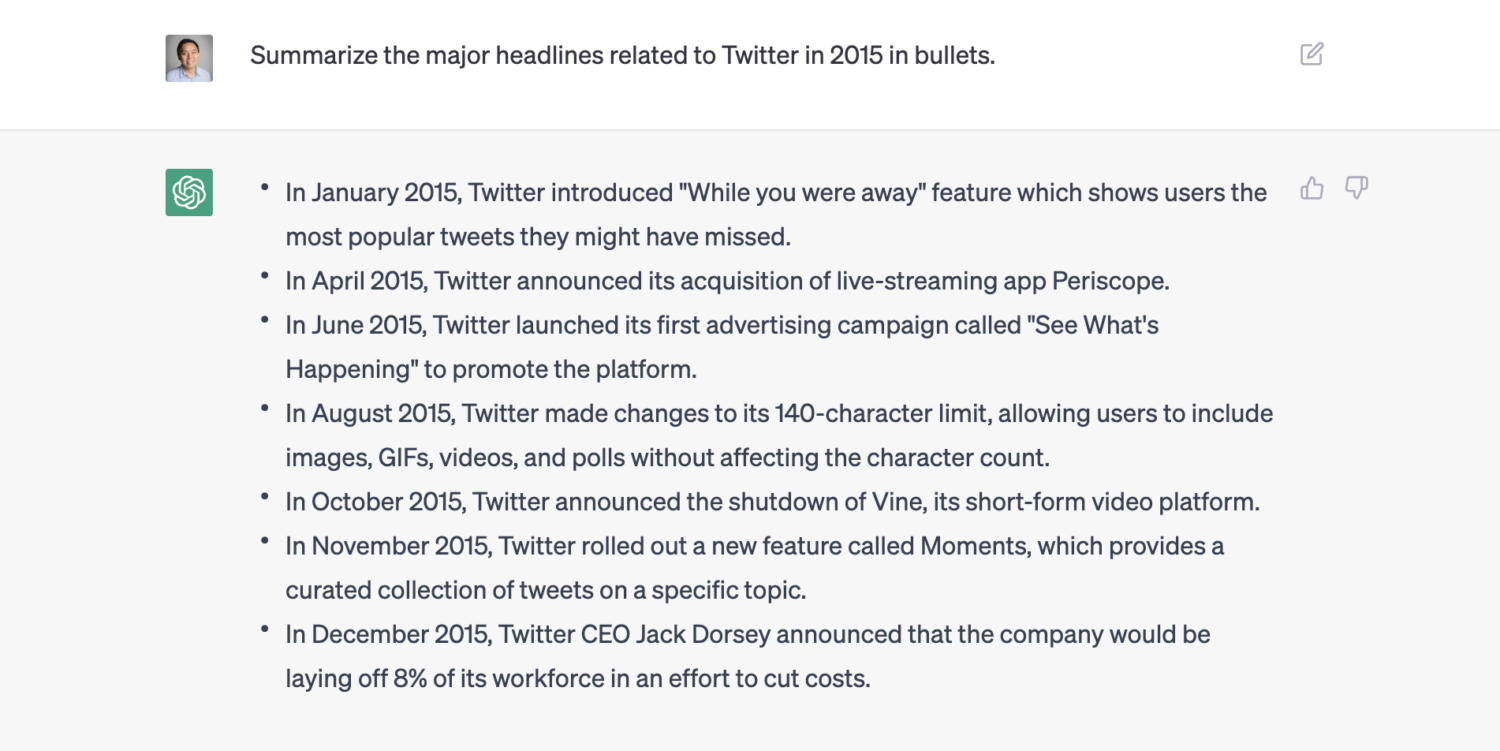
Oho! That actually looks pretty good. In fact, all of these headlines are real events, but the dates are garbage. 1 of them is correct, 3 off by a year, and 3 off by some months. Here’s how it breaks down (sources linked) —
- In January 2015, Twitter introduced “While you were away” feature which shows users the most popular tweets they might have missed.
- In
AprilJanuary/February 2015, Twitter announced its acquisition of live-streaming app Periscope. - In
June 2015July 2016, Twitter launched its first advertising campaign called “See What’s Happening” to promote the platform. - In
August 2015May 2016, Twitter made changes to its 140-character limit, allowing users to include images, GIFs, videos, and polls without affecting the character count. (Twitter did make changes on the character limit in August 2015, but it was specifically to DMs.) - In
October 2015October 2016, Twitter announced the shutdown of Vine, its short-form video platform. - In
NovemberOctober 2015, Twitter rolled out a new feature called Moments, which provides a curated collection of tweets on a specific topic. - In
DecemberOctober 2015, Twitter CEO Jack Dorsey announced that the company would be laying off 8% of its workforce in an effort to cut costs.
I don’t think Dr. Hartdegen would be impressed. But this isn’t breaking news, many of us are well aware by now that ChatGPT makes stuff up and isn’t knowledgeable of events beyond 2021.
In this prompt study, I constrain GPT’s fact recall to a trusted news graph, and take advantage of its language transformation capabilities to cluster and generate top line summaries of similar events. Response output is also formatted in JSON, making it easy to plug into data pipelines.
The technique can be applied to both point-in-time media research or real time monitoring. I will demonstrate how to do both.
ChatGPT talks to a knowledge graph
A common misunderstanding for ChatGPT’s lack of current event knowledge is that it lacks training from recent news.
While technically true, further training only serves to reinforce word patterns in its model, which bears the same limitations (lack of provenance and inaccurate dates) as the events it attempted to retrieve on the Twitter example above.
Instead, we will supply recent knowledge in the prompt, which will also enable GPT to understand and act on it structurally (e.g. citing from the corpus)
Let’s try to figure out what happened at Twitter in 2015 again. This time, we will provide a sample of 50 headlines mentioning Twitter in 2015, sourced from the Diffbot Knowledge Graph. Here is the DQL used to pull this sample:
type:Article title:'Twitter' categories.name:'Business' tags.{label:'Twitter' score>0.95} language:'en' date>="2015-01-01" date<="2015-12-31" sortBy:date
We’ll want to format the response as CSV and request the date and headline fields. Plug the CSV results of this query into a prompt template as follows:
The following is a list of headlines related to Twitter each with a date attached. Generate a list of the top 5 things that happened at Twitter based on these headlines alone. Use the following forma for each item on the list: On <March 11, 2015>, <summary of what happened>. When reviewing these headlines, ignore stories, gossip, editorials, opinions, politics, or any headlines not related to a decision or action made by Twitter the company. Focus only on headlines that could exist on a Twitter press release. Do not hallucinate. Order the list by earliest to latest. 2015-03-11 Twitter updates its rules to specifically ban ‘revenge porn’ 2015-01-07 The Story of Twitter's Fail Whale 2015-11-23 Bezos tweets! Twitter feud with Warren Buffett next? 2015-06-11 Twitter's Dick Costolo (briefly) got richer by quitting 2015-10-04 Twitter names Jack Dorsey as CEO 2015-06-06 Here's an Android app that gives people in censored countries access to Twitter 2015-11-02 Twitter ditches stars and favorites for hearts and likes 2015-10-05 Twitter Names Co-Founder Jack Dorsey CEO 2015-10-13 Why Twitter Is Laying Off 8 Percent of Its Employees 2015-03-26 Twitter's Periscope Live Streaming App Makes Everyone a Reality Star 2015-12-21 How Jack Dorsey Runs Both Twitter, Square 2015-07-26 When will Twitter name a new CEO? 2015-09-15 Twitter Courts U.S. Presidential Campaigns With New Donations Service 2015-11-03 Inside Twitter's big diversity problem 2015-06-11 Twitter (TWTR) CEO Dick Costolo Stepping Down 2015-07-21 Twitter throws frat-themed party in midst of discrimination suit 2015-06-22 Twitter Says Its New Chief Must Work Full Time 2015-12-17 Twitter blows up over Martin Shkreli's arrest 2015-08-09 #Touchdown! NFL partners with Twitter 2015-09-02 Twitter could name its new CEO today 2015-07-11 Twitter Accidentally Made Scott Walker a Presidential Candidate Ahead of Schedule 2015-10-13 Twitter just hired Google's $130 million man 2015-10-26 Twitter still hasn't found its groove - stock tanks 2015-10-06 Saudi prince now owns 5% of Twitter 2015-07-27 Conan O'Brien accused of stealing jokes from Twitter 2015-10-05 Jack Dorsey Will Return As Twitter CEO 2015-08-19 #EpicFail: Twitter falls below $26 IPO price 2015-07-13 Twitter shares soar on phony Bloomberg story 2015-03-09 Twitter Acquires Live-Video Streaming Startup Periscope 2015-01-26 Twitter Chat on the Internet of Things 2015-03-12 Twitter bans 'revenge porn' 2015-06-03 Big Twitter investor Chris Sacca explains what the company needs to do next 2015-10-05 IT'S OFFICIAL: TWITTER MAKES JACK DORSEY FULL-TIME CEO 2015-06-01 A Twitter bot has spent the entire day scolding people who are talking about Caitlyn Jenner 2015-02-05 Twitter’s battle against abuse has high stakes for the company 2015-01-15 New York Post and UPI Twitter accounts hacked 2015-06-11 For Twitter, Future Means Here and Now 2015-07-26 Kim Kardashian tweets and Twitter's boss listens 2015-04-28 Twitter Shares Tumble After Nasdaq Leaks Results Early 2015-09-18 Twitter Helps Coke With Ad ‘Emoji’ 2015-10-12 Twitter cuts 8% of its workforce 2015-03-01 ISIS supporters threaten Twitter founder Jack Dorsey 2015-12-15 Twitter is too cool for school. It must go mainstream 2015-07-31 James Woods Sues Twitter Troll for $10 Million Over ‘Cocaine Addict’ Tweet 2015-10-14 The 129 finance people you have to follow on Twitter 2015-04-02 Chelsea Manning joins Twitter and gets over 1,000 followers before posting 2015-10-12 Did the NFL kill Twitter accounts for Deadspin, SB Nation? 2015-04-28 Will Dick Costolo's Twitter Keep Disappointing Investors? 2015-06-10 Twitter CEO Dick Costolo quits 2015-02-17 Boss Talk: Twitter Safety Officer Talks Building Trust
This is GPT-3.5’s response:
Based on the provided headlines, here are the top 5 things that happened at Twitter: 1. On March 11, 2015, Twitter updated its rules to specifically ban 'revenge porn.' 2. On June 11, 2015, Twitter CEO Dick Costolo announced his resignation. 3. On October 4, 2015, Twitter named Jack Dorsey as CEO. 4. On October 5, 2015, it was officially confirmed that Jack Dorsey would be the full-time CEO of Twitter. 5. On November 2, 2015, Twitter replaced stars and favorites with hearts and likes. Note: The list is ordered chronologically based on the headlines.
A great start. It was at this point where I proceeded to spend two frustrating weeks getting ChatGPT to actually cite from my provided sources, a surprisingly complicated task.
I’ll spare you the bitter details of dead ends and square ones. Instead, here is what I learned:
- Instruction order matters. If you are providing context, state this before dropping in the context. Always end with instructions.
- ChatGPT will error with too much text in the prompt. A rookie mistake. Don’t stuff your prompt with thousands of headlines. More below.
- ChatGPT will ignore your instructions if there is too much context. Even if your prompt is within the token limit, providing too much context in your prompt will cause ChatGPT to ignore your instructions. I’ve found the sweet spot to be roughly 60 headlines.
- Lose the URLs. Indexes or IDs work better. URLs in your context dump will just take up precious token space. ID your sources to save on tokens.
Here is the prompt that finally got ChatGPT to cite from my provided headlines:
The following is the CSV output of a search for articles on the company FTX:
title,id,date.timestamp
FTX collapse: Black Swan really happen!,ART195719715414,1668614400000
"FTX did not have permission to take on Dutch clients, says central bank",ART6137214573,1668614760000
<...and 58 more headlines>
Based on the above headlines, summarize 1-4 major events that happened at FTX in a JSON array of events with each event having a key name, date (in its original epoch time date format), and a JSON array of citationIds to the relevant articles. Cluster similar headlines to the same event, even if they may not occur on the same date. If they do not have the same date, use the earliest known date. Ignore headlines about FTX's stock price, stock performance, dividends, or market performance. Also ignore headlines about gossip, social commentaries, thoughts, potentials, explorations, hearsay, maybes, and opinions. Summaries should include a verb. Only return the JSON, no further text or explanation, do not use markdown.
With this prompt, ChatGPT returns the following response:
[
{
"name": "FTX Collapse",
"date": 1668614400000,
"citationIds": ["ART195719715414"]
},
{
"name": "FTX Did Not Have Permission to Serve Dutch Clients",
"date": 1668614760000,
"citationIds": ["ART6137214573"]
},
{
"name": "Celebrities Named in FTX Suit",
"date": 1668615900000,
"citationIds": ["ART257680608131"]
},
{
"name": "FTX's Bankruptcy Explained",
"date": 1668616440000,
"citationIds": ["ART226107828117"]
},
{
"name": "Billionaire Mike Novogratz Warns of More Failures",
"date": 1668617820000,
"citationIds": ["ART149839769712"]
},
{
"name": "Coinbase CFO Anticipates Contagion Impact of FTX Collapse",
"date": 1668620400000,
"citationIds": ["ART127028717456", "ART246501061069"]
},
{
"name": "FTX Investors Sue Bankman-Fried Over Yield-Bearing Crypto Accounts",
"date": 1668621600000,
"citationIds": ["ART237167380194"]
},
{
"name": "Relief Fund Established for Partners Affected by FTX Collapse",
"date": 1668623761000,
"citationIds": ["ART76130626106", "ART211198078567"]
},
{
"name": "FTX Management and Bahamas in Legal Battle Over Bankruptcy Control",
"date": 1668628260000,
"citationIds": ["ART112350911287"]
},
{
"name": "Former SEC Official Doubts FTX Crash Will Lead to Crypto Regulations",
"date": 1668624600000,
"citationIds": ["ART35623621602"]
}
]
This prompt transforms 60 raw headlines to 10 top line summaries citing real news article sources without hallucinations. It also formats the response into a JSON array! We finally have our working proof of concept.
This is our boilerplate prompt. From here, we can also filter the output to specific types of events.
Traditionally this would mean setting up complex layers of string filters and even classification models to identify different types of articles. With GPT, we can simply tune the instruction with plain English to highlight only the events we care about.
Let’s take it back to Twitter and focus on top lines from the last week.
First, tweak the DQL to query for headlines in the news within the last 7 days.
type:Article title:'Twitter' categories.name:'Business' tags.{label:'Twitter' score>0.95} language:'en' date<=7d sortBy:date
Then we’ll adjust the instruction of the prompt to only top line revenue related headlines on Twitter. Here is the new instruction snippet and corresponding response:
Based on the above headlines, summarize any major events that happened at Twitter in an array of JSONs with each JSON item having a key name, date (in its original epoch time date format), and a JSON array of citationIds to the relevant articles. Cluster similar headlines to the same event, even if they may not occur on the same date. If they do not have the same date, use the earliest known date. Only summarize headlines directly related to revenue. If there are no headlines related to revenue, return an empty array. Summaries should include a verb. Only return the JSON, no further text or explanation, do not use markdown.
[
{
"name": "Twitter's ad revenue plummets",
"date": 1685990580000,
"citationIds": [
"ART172232690543",
"ART21141493577",
"ART141839195523"
]
},
{
"name": "Twitter bans over 25 lakh accounts in India",
"date": 1685685720000,
"citationIds": [
"ART184478583226"
]
},
{
"name": "Twitter Value Collapses After Fidelity Writedown",
"date": 1685634000000,
"citationIds": [
"ART9548418736"
]
}
]
How about something trickier? Like politics?
Based on the above headlines, summarize any major events that happened at Twitter in an array of JSONs with each JSON item having a key name, date (in its original epoch time date format), and a JSON array of citationIds to the relevant articles. Cluster similar headlines to the same event, even if they may not occur on the same date. If they do not have the same date, use the earliest known date. Only summarize headlines directly related to politics. If there are no headlines related to politics, return an empty array. Summaries should include a verb. Only return the JSON, no further text or explanation, do not use markdown.
[
{
"name": "Twitter's Disagreement with EU on Disinformation Code",
"date": 1686061842000,
"citationIds": [
"ART51264025820"
]
},
{
"name": "Senators Warning Twitter and Elon Musk over Data Privacy",
"date": 1685951340000,
"citationIds": [
"ART144302563391"
]
}
]
One more go, this time we’ll give it a curveball.
Based on the above headlines, summarize any major events that happened at Twitter in an array of JSONs with each JSON item having a key name, date (in its original epoch time date format), and a JSON array of citationIds to the relevant articles. Cluster similar headlines to the same event, even if they may not occur on the same date. If they do not have the same date, use the earliest known date. Only summarize headlines directly related to sushi. If there are no headlines related to sushi, return an empty array. Summaries should include a verb. Only return the JSON, no further text or explanation, do not use markdown.
[]
Being explicit is important here. ChatGPT loves to please. Without the option to return an empty array, ChatGPT becomes highly unpredictable. In my testing, I got it to hallucinate sushi related headlines while simultaneously informing me that there are no sushi related headlines.
How do I use this?
Check out the Github repo for some code examples in Python that can be easily translated into a news monitoring workflow.
Not a developer? Stay tuned for a feature I’m building in LeadGraph that uses this technique to summarize and highlight the latest headlines from your target accounts.
Bonus: Examining the rise and fall of FTX
If we can reliably summarize the top lines from a blob of 60 headlines, what would it look like if we ran this prompt across all known articles on a company like FTX?
I hoped to generate something close to the timeline walls you see in history museums.
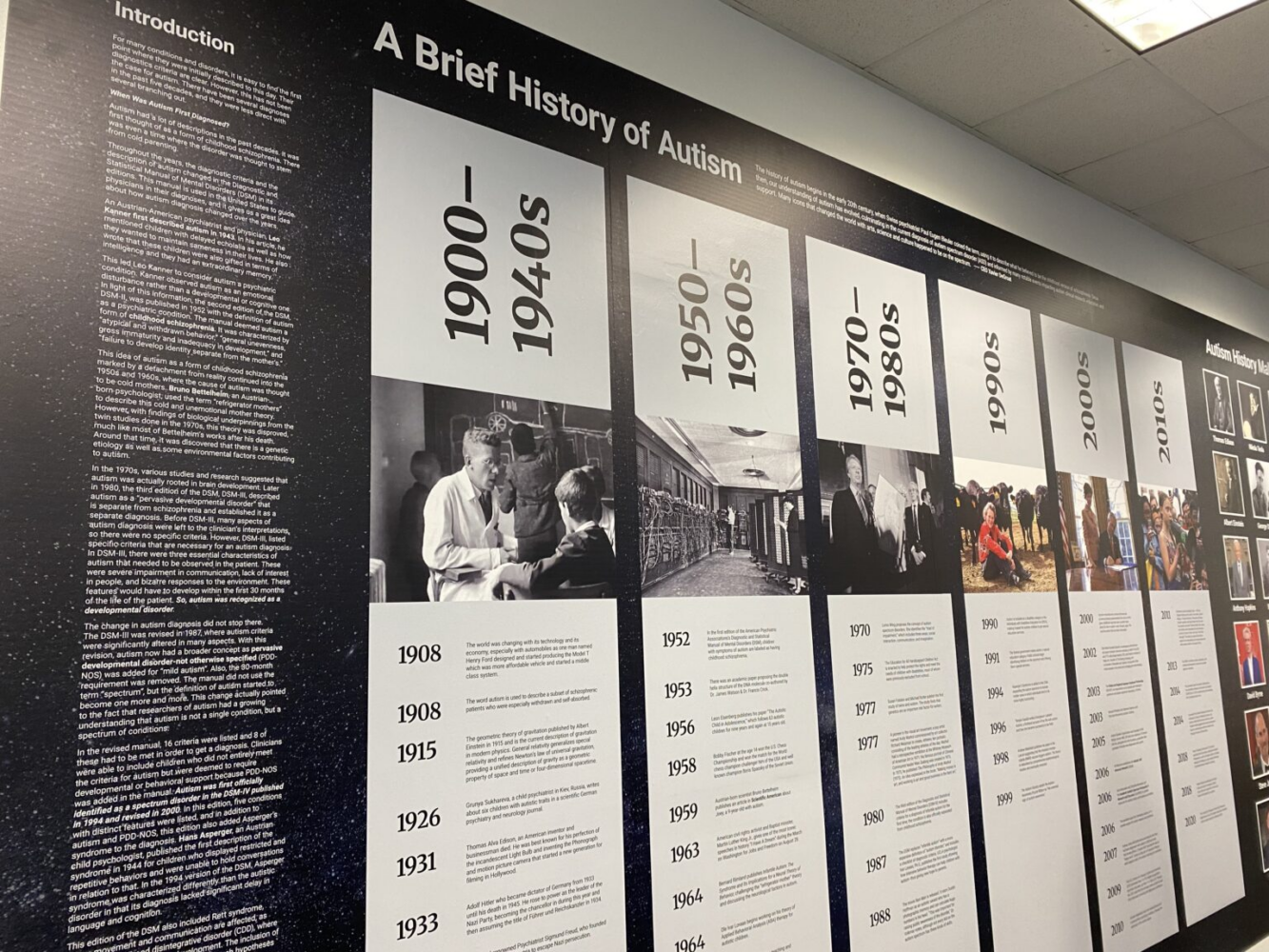
And boy did I.
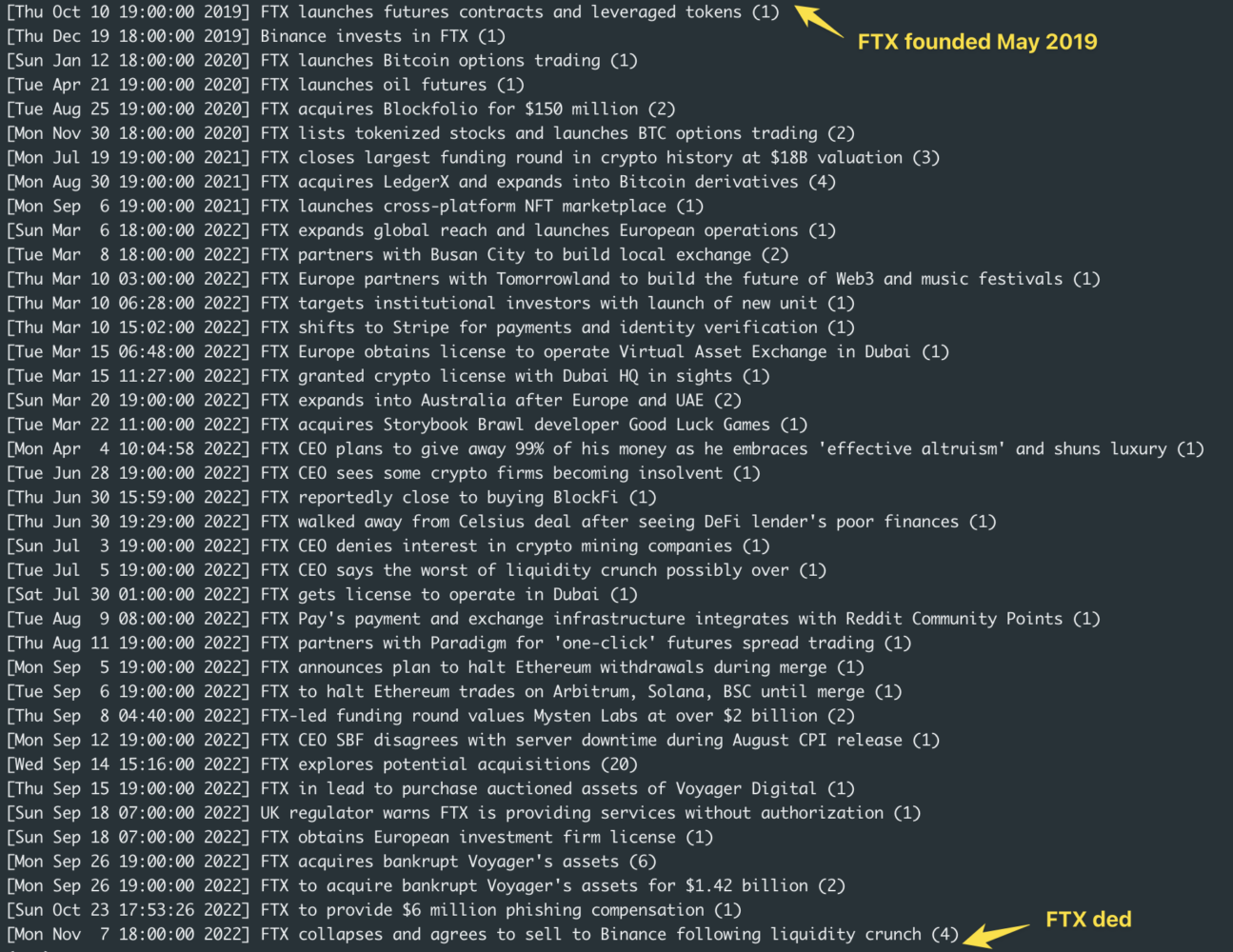
The script takes a single input – the name of an organization – and summarizes the major events within blobs of headlines. Here are the high level order of operations:
- Enhance the org name with Diffbot KG to obtain a
foundingDate
- Use the
foundingDate
as a start date in our Diffbot News Graph article query mentioning the company (60 at a time) - Plug the 60 headlines into a request to the chat completions OpenAI endpoint using the
gpt-3.5-turbo
model - Write GPT’s JSON response into a jsonl file
- Loop steps 2-4 until there are no articles left
The same Github repo includes a generate_timeline.py
Python script to reproduce this yourself. You will need an OpenAI API token as well as a Diffbot token. A warning — processing 60 headlines at a time takes awhile, but the results are stunning.
You must be logged in to post a comment.