Skip Ahead
2020 was undeniably the “Year of the Knowledge Graph.”
2020 was the year that Gartner put Knowledge Graphs at the peak of its hype cycle.
It was the year where 10% of the papers published at EMNLP referenced “knowledge” in their titles.
It was the year over 1000 engineers, enterprise users, and academics came together to talk about Knowledge Graphs at the 2nd Knowledge Graph Conference.
There are good reasons for this grass-roots trend, as it isn’t any one company that is pushing this trend (ahem, I’m looking at you, Cognitive Computing), but rather a broad coalition of academics, industry vertical practitioners, and enterprise users that generally deal with building intelligent information systems.
Knowledge graphs represent the best of how we hope the “next step” of AI looks like: intelligent systems that aren’t black boxes, but are explainable, that are grounded in the same real-world entities as us humans, and are able to exchange knowledge with us with precise common vocabularies. It’s no coinincidence that in the same year that marked the peak of the deep learning revolution (2012), Google introduced the Google Knowledge Graph as a way to provide interpretability to its otherwise opaque search ranking algorithms.
The Risk Of Hype: Touted Benefits Don’t Materialize
However, like all trends, there is great risk of not living up to the hype, and as the Gartner Hype cycle suggests, this risk is almost an inevitability if one subscribes to cyclical models of innovation. So it feels like almost a truism to state that the hyped benefits won’t materialize.
A lot of focus of the hype so far, has been around touting the benefits of organizing your organization’s data into some sort of centralized graph structure. If the hype is to be believed, having a central Knowledge graph will unify all of your disparate data silos, power your complex business decisions, and “future-proof” your data. Because standards… Also, it’s used at FAANG companies, so it must be good (nevermind the fact that they aren’t actually using KGs to organize their internal documents).
Sure, being able to find all your data in your org sounds like a good idea (see: Google). But is centralizing the engineering team’s database and the sales team’s database something either team wants? Is adopting a common vocabulary across the organization going to “future-proof” your data? Or is it going to create endless meetings where updates for vocabularies and ontologies are discussed?
One thing that is certain is that you will need a whole stack of vendors and consultants to help you undergo this digital transformation and it will take an upfront investment in time and money. You will definitely need a graph database, an ontology, and to train everyone on this new graph query language as well as in using a new visualization tools stack.
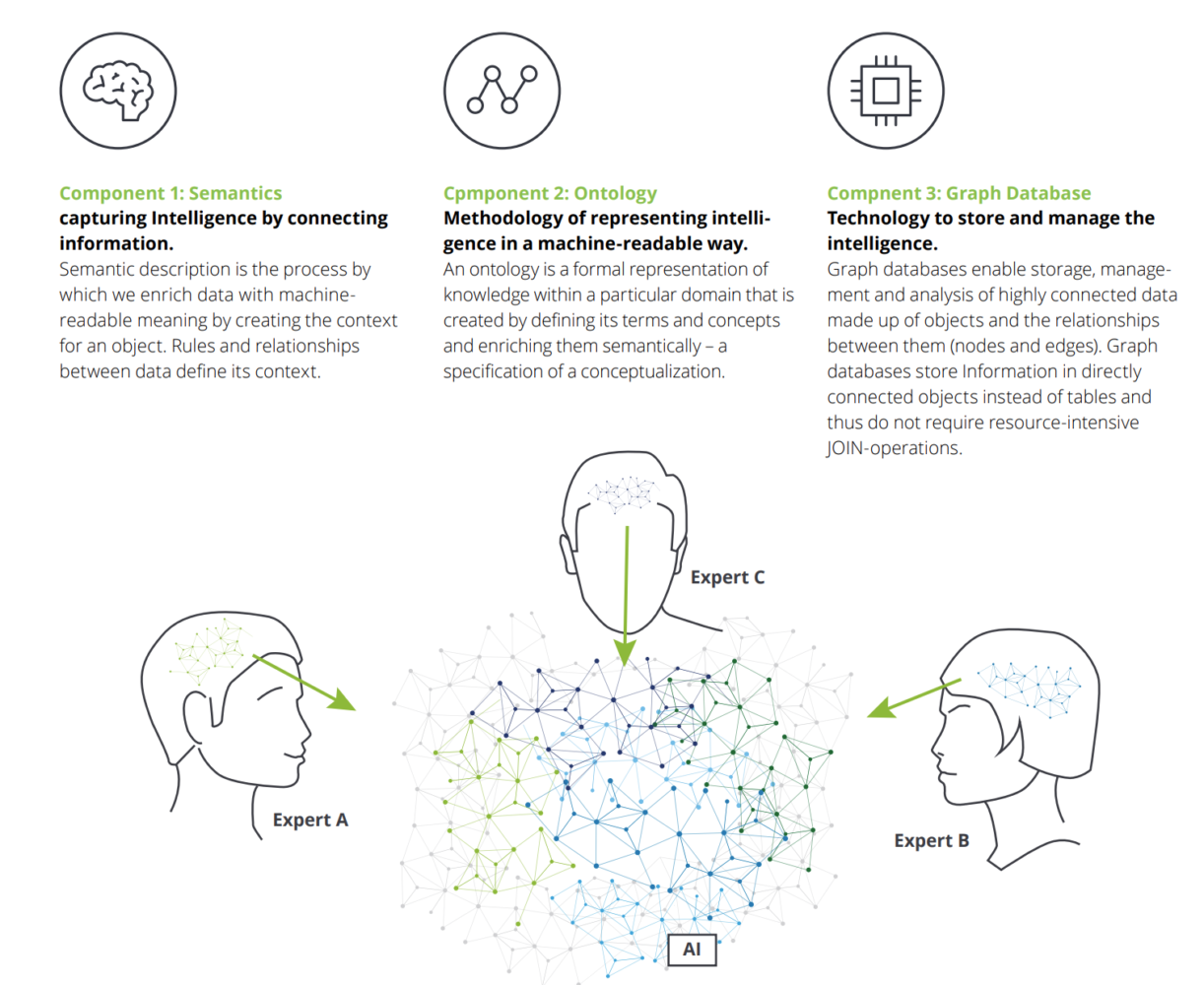
We’ve Seen This before
If that sounds a tad bit insane, perhaps the larger collective insanity is that we’ve seen this story play out before and yet we’re expecting a different outcome this time.
Tim Berners-Lee’s concept of the Semantic Web was also supposed to herald a new form of information exchange that would enable new kinds of intelligent applications. While semantic web technologies have had a fruitful life in focused verticals (supply-chain management and oil & gas come to mind), those ideas haven’t worked as a means for widespread information exchange across a diverse set of human uses (like the open web).
We risk seeing the same semantic web story play out again within the battlefield of the corporate intranet. After all, a company is simply a microcosm of larger global society–a non-homogenous group of people with diverse interests, and specialized teams. Cory Doctorow’s harsh critique of the semantic web technologies largely applies to the humans of the corporate intranet as well.
A Simpler Statement Of The Benefits Of KG tech
I want to propose a much simpler statement of the benefits of knowledge technologies. KG technologies are indeed useful, but the most productive use of them is not in building a centralized enterprise knowledge graph. We shouldn’t be fixated on the graph itself, but on enabling knowledge workflows.
Knowledge Graph technologies allow the introduction of automation into information worker workflows, helping them save time on mundane information processing tasks.
That’s it.
It’s simply a way to introduce automation to the person who’s job it is to process a lot of incoming information by making that information incrementally more machine readable.
That person could be a sales rep, a project manager, a marketer, a bookkeeper, a recruiter, or a teacher. We haven’t even begun to think about all of the different workflows that KG technologies can improve in the systems information workers use.
KG technologies do this by taking the most mundane, high frequency, high volume information processing tasks and by transforming parts of it into a machine parseable representation. This allows for the automated implementation of business logic.
The core of Knowledge technologies is the structuring of information such that it is machine understandable. Knowledge tech != RDF, using a graph DB, or adopting specific vendors.
By freeing ourselves of these old notions of what knowledge technologies are, we can embrace a much wider set of workflows and have greater impact as an industry. There is no need to undertake large digital transformation projects that take months to years when we can have real impact to someone’s daily workflow immediately.
Having a large centralized knowledge graph is actually not a net asset but a liability–not only because it serves as a central point of security breach, but because having any large set of data in one place causes it to go stale as the world that data reflects changes. We should think of large collections of internal data like physical inventory, there is a depreciation and warehousing cost. A much better model is to have federated, distributed workflows that each team owns, keeping small amounts of internal state and tapping into automatically updated streams of external data to get the latest information at the point in time its used.
Take Away
For knowledge graph technologies to have a broader impact, we shouldn’t be dogmatic about a term that was invented by Google’s product marketing department. We shouldn’t insist on adoption of standardized markup schemes or the creation of a centralized graph database because that didn’t work for the Semantic Web, and it won’t work for the corporate web.
If we instead listen to the problems information workers have, spend a day shadowing them in their jobs, and design solutions that integrate knowledge-tech in a lightweight way to automate tedium, then we have a shot at solving a larger set of problems, to benefit more of society.
You must be logged in to post a comment.